Artificial Intelligence's Impact on Stock Trading
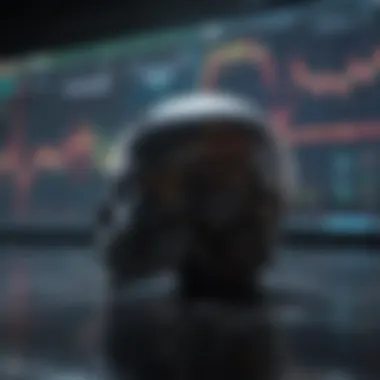
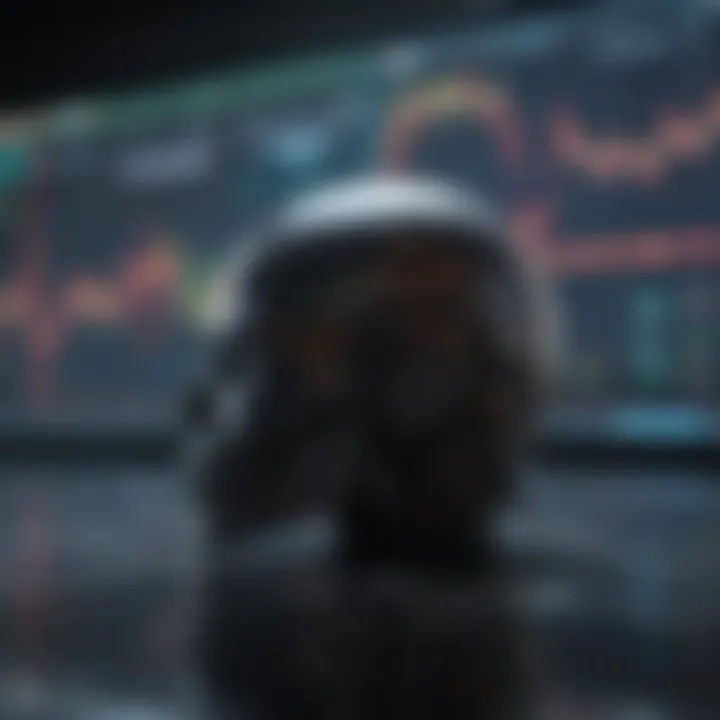
Intro
Artificial Intelligence is making waves across various sectors, and the stock market is no exception. With deep learning algorithms and machine learning models evolving rapidly, the financial world is seeing a transformation that's hard to ignore. From analyzing vast amounts of data to predicting market trends, AI is reshaping not just how trades are conducted, but also how investments are managed and assessed.
This article will delve into how these technologies are being utilized in finance. We're going to explore key terms and concepts that lay the groundwork for understanding AI's influence. Then, we'll dive into investment strategies, considering how AI can enhance decision-making for both long-term and short-term investors. Finally, we will touch on ethical considerations and future trends that are crucial as we move forward in this AI-driven market.
Key Terms and Concepts
To navigate the evolving landscape created by AI in finance, it is essential to understand certain key terms. These terms provide clarity and insight into how AI operates within the stock market.
Definition of Key Terms
- Artificial Intelligence (AI): Refers to the simulation of human intelligence in machines that are programmed to think and learn. This includes techniques such as machine learning and deep learning.
- Machine Learning: A subset of AI focused on teaching machines to learn from data. It empowers algorithms to make predictions or decisions without being explicitly programmed for each task.
- Natural Language Processing (NLP): This is the ability of AI to understand, interpret, and respond to human language in a valuable manner. In finance, NLP analyzes news articles, earnings reports, and social media to gauge market sentiment.
Importance of Understanding Terminology
Understanding these terms is crucial because they form the backbone of discussions around AI in the investing world. For instance, recognizing how machine learning can analyze historical stock data allows investors to grasp the potential benefits and limitations of algorithmic trading. Similarly, understanding NLP can aid in leveraging news for investment decisions.
Investment Strategies
With AI's integration into investment strategies, both novice and seasoned investors can refine their approaches to trading. The technology not only augments existing methods but also introduces new ways to evaluate risk and optimize portfolios.
Long-term vs. Short-term Investment
- Long-term Investment: Involves holding securities for an extended period, focusing on fundamental analysis, where AI can help identify undervalued stocks by analyzing company performance metrics and market conditions. Investors may use predictive analytics to forecast long-term growth potential.
- Short-term Investment: This strategy focuses on taking advantage of short-lived market fluctuations. Here, AI can enhance trading algorithms to respond quickly to market signals, improving the timing of trades and potentially increasing profits.
Risk Tolerance and Asset Allocation
Investors need to define their risk tolerance when implementing AI-driven strategies. AI can facilitate a more tailored approach to asset allocation, allowing investors to diversify their portfolios based on individual risk profiles.
"Investment trends are shifting fast; those who understand AI's role have a leg up in adjusting their strategies effectively."
For example, an investor with a lower risk tolerance may benefit from AI systems that balance portfolios with more stable assets, while aggressive investors might leverage AI tools for identifying high-risk opportunities in volatile markets.
By grasping these core concepts and effectively utilizing AI tools, investors can stay ahead in the rapidly evolving financial landscape. The intersection of technology and stock trading represents a shift that has the potential to revolutionize investment strategies for the foreseeable future.
Preamble to Artificial Intelligence in Finance
Artificial Intelligence (AI) has become a buzzword in many fields, but its intersection with finance is particularly compelling. The financial world, known for its complexities, volatility, and unpredictable nature, has seen AI emerge as a game-changer. This section aims to illuminate the importance of AI in finance by exploring its fundamentals and shedding light on its historical significance.
Defining Artificial Intelligence
To understand how AI fits into finance, itās essential to first define what it is. In straightforward terms, AI refers to the capability of a machine to imitate intelligent human behavior. This includes learning from experiences, adapting to new inputs, and performing tasks that typically require human intelligence. In finance, AI encompasses a spectrum of technologies like machine learning, neural networks, and natural language processing.
Many financial institutions are adopting AI to streamline operations, enhance decision-making, and improve client interactions. With the potential to analyze vast amounts of data swiftly, AI can sift through millions of transactions to find patterns that human analysts might overlook. By using sophisticated algorithms, it can also predict market trends and automate trading processes.
āIn the world of finance, the ability to analyze data efficiently and accurately can make the difference between profit and loss.ā
Historical Context of AI in Finance
The story of AI in finance is not a recent one. It began gaining ground in the late 20th century when computers entered the financial landscape. Initially, quantitative analysts and mathematicians started using simple algorithms to solve complex financial problems. These early attempts laid the groundwork for the evolution of more advanced AI applications today.
In the 1980s and 1990s, the introduction of neural networks provided a significant leap forward. These systems were mimicking the way human brains operate, allowing for more sophisticated data processing and prediction capabilities. However, it wasnāt until the 2000s that AI started making a real impact on trading platforms, enabling high-frequency trading, where decisions were executed in milliseconds based on algorithmic analysis.
With the rise of big data in the 2010s, AI took another giant leap. Access to varied datasets allowed financial institutions to refine algorithms, providing deeper insights into market dynamics. Now, AI is not just about making tradesāitās about comprehending the intricate landscape of financial markets, analyzing sentiment through news sources, and even forecasting economic conditions based on real-time data.
In summary, AI's role in finance has evolved from basic computations to advanced predictive models, making it an indispensable tool for todayās investors and financial professionals.
Applications of AI in Stock Trading
Artificial Intelligence has forged its way into the heart of stock trading, revolutionizing how market participants operate. By enabling faster, more precise decision-making, AI applications provide significant advantages, enhancing efficiency and accuracy in trading strategies.
The intersection of AI and stock trading is vital for todayās investors due to its multifaceted nature, incorporating different methodologies that cater to diverse aspects of trading. From algorithmic trading that executes trades at lightning speed to advanced market prediction techniques that leverage extensive data sets, AI stands at the cusp of redefining how investments are managed and evaluated. The transformative impact of AI not only caters to seasoned investors looking to refine their strategies but also beginners who can leverage sophisticated tools to navigate the complexities of the market.
Algorithmic Trading
Algorithmic trading refers to a set of automated strategies designed to execute trades at optimal prices during market hours. This approach harnesses the power of technology to analyze vast amounts of data, resulting in quicker and more informed trading decisions. Itās not just about speed; it's also about using sophisticated models divorced from human emotion, making it an increasingly popular choice for traders.

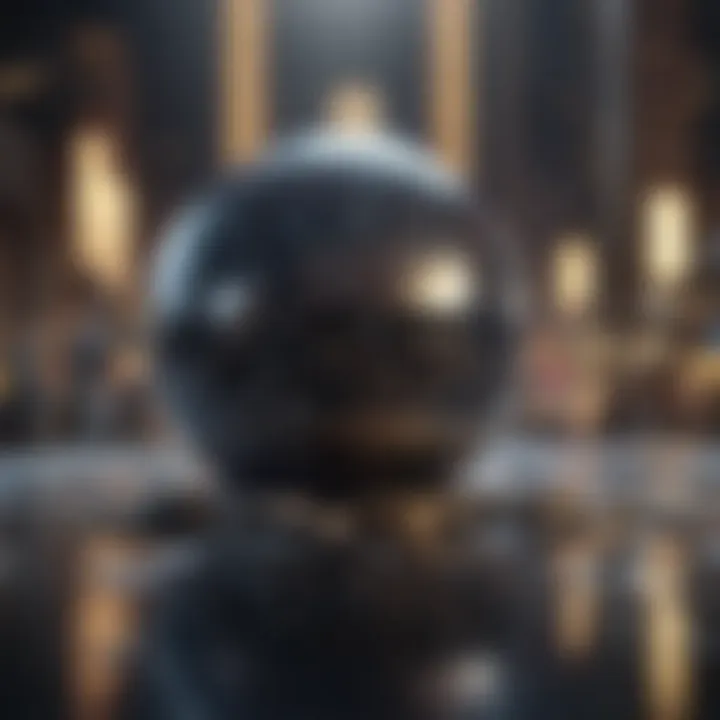
High-Frequency Trading
High-frequency trading (HFT) exemplifies the cutting-edge capabilities of algorithmic trading. It relies heavily on algorithms to execute orders within fractions of a second, taking advantage of minute price discrepancies in the market. What makes HFT particularly attractive is its capacity to generate substantial profits over numerous trades, often leveraging sizable market volumes.
However, there are downsides. The high transaction costs and risks associated with getting tangled in market volatility present challenges. The technology demands significant investment and expertise, making it more suitable for institutional investors rather than individual traders. Nevertheless, for those who can manage the infrastructure, HFT can yield impressive returns.
Quantitative Strategies
Quantitative strategies lean on statistical models and mathematical techniques to identify trading opportunities and forecast market movements. These strategies analyze historical data to make predictions about future price changes. Investors favor quantitative approaches due to their reliance on data-driven insights instead of subjective analysis.
Such strategies can adapt quickly to new information, which is essential in the fast-paced stock trading environment. However, a reliance on quantitative models carries its risks. If the underlying assumptions of a strategy do not hold true in future market conditions, it can lead to undesired outcomes. Still, for those adept in mathematics and statistics, quantitative strategies represent a powerful avenue to potentially enhance investment returns.
Market Prediction and Analysis
Understanding market trends and predicting future price movements is crucial in successful trading. AI has introduced remarkable advancements in this area, employing data analysis techniques that significantly improve predictions.
Machine Learning Techniques
Machine learning techniques deploy algorithms that learn from historical data to identify patterns and trends. This approach can predict stock price movements by analyzing various factors, such as trading volumes, price trends, and even social media sentiments.
By learning from past data, machine learning can create more refined and accurate predictive models. However, the complexity of these algorithms means that they require large data sets for effective training, which might not always be available. Furthermore, the models can also 'overfit' the data, leading to erroneous predictions. Still, these techniques are becoming indispensable in crafting successful trading strategies.
Sentiment Analysis
Sentiment analysis takes a different approach by gauging the public sentiment around particular stocks or the market in general. By analyzing social media chatter, news articles, and forums, AI tools can provide insights into how market sentiment influences stock prices.
The beauty of sentiment analysis lies in its ability to quantify otherwise subjective opinions, allowing traders to anticipate market movements based on public perception. However, itās not an all-encompassing solution; sudden shifts in sentiment can occur due to external news shocks or events, leading to unpredictable market reactions. Nevertheless, sentiment analysis equips traders with an additional lens through which to view market conditions and dynamics.
"AI applications in stock trading present a unique intersection of complex data analytics and real-world financial strategies, pushing the boundaries of what is possible in investment management."
In summary, the integration of AI into stock trading has birthed myriad tools and strategies that enhance decision-making, reduce emotional bias, and exploit market inefficiencies with unparalleled speed. As these technologies evolve, they promise to reshape the investment landscape, making them essential for both new and seasoned investors.
The Role of Machine Learning in Pricing Models
In the intricate world of financial markets, pricing models serve as the backbone for investment decisions. By harnessing the power of machine learning, these models are continually evolving to become more accurate and efficient. The transition from traditional pricing methods to those powered by machine learning has opened doors to deeper insights and predictive capabilities, reshaping the way investors and analysts approach the market.
Understanding Pricing Models
Pricing models are mathematical frameworks utilized to determine the theoretical fair value of assets. Typically, these models are built on fundamental financial theories and have been used for decades in the financial industry. However, as the markets grew complex, the limitations of traditional models became evident. Machine learning offers a refreshing change by introducing algorithms that can learn from vast datasets and adapt to new information swiftly.
For instance, consider the Black-Scholes model, a classic in options pricing. While it provides a solid foundation, it doesn't account for market anomalies and behavioral factors. In contrast, a machine learning-driven model can incorporate an array of variablesālike historical pricing data, market sentiment, and economic indicatorsāallowing for dynamic adjustments in real time. This adaptability can lead to better pricing accuracy, especially in volatile market conditions.
Additionally, the application of machine learning in pricing models can enhance predictive abilities. By using historical data to train models, machine learning algorithms can discern patterns that might be invisible to human analysts. This predictive edge can be crucial, especially when trying to anticipate rapid price changes in response to economic news or geopolitical events.
Data Processing and Feature Selection
When it comes to machine learning, data serves as the lifeblood. Effective data processing is paramount for accurate pricing models. This involves cleaning the data, handling missing values, and selecting relevant features that can significantly impact asset prices. The process can often be akin to mining for gold; you need to sift through a lot of dirt to find valuable insights.
Feature selection, a core aspect of the model-building process, determines which variables should be included in the model. This step is critical because not every available piece of data is beneficial for pricing. The goal is to identify features that have predictive power without introducing unnecessary noise. For example, rather than considering every economic indicator available, a model might hone in on a select fewālike unemployment rates or consumer sentimentāwhich have a proven correlation with market movements.
As a case in point, a model could utilize data from social media platforms to gauge public sentiment about a particular stock. By combining this qualitative data with quantitative metrics, analysts can build a more holistic view of market conditions.
"Incorporating machine learning into financial pricing models is not just about complexity; itās about developing a sense of market intuition that was hard to achieve with classic methods."
Embracing machine learning in pricing models leads to innovative approaches in finance. The potential for increased accuracy in pricing and improved risk management cannot be overstated. As investors and market analysts continue to seek a competitive edge, understanding and adopting these advanced methodologies becomes crucial in navigating the ever-evolving stock market.
Natural Language Processing in Financial News
Natural Language Processing (NLP) plays a pivotal role in the intersection of finance and technology, especially in how financial news is analyzed and interpreted. In todayās fast-paced market, the confluence of data and language is invaluable. Investors and market analysts find themselves swimming in an ocean of information, where real-time analysis can make or break investment decisions. Therefore, deploying NLP not only streamlines the clutter but elucidates patterns hidden within texts, offering insights that human analysts might overlook.
Through machine learning algorithms, NLP systems can dissect vast quantities of financial news articles, press releases, and social media chatter to gauge the overall sentiment surrounding a stock or a sector. This capability equips investors with almost instantaneous feedback on market perception, allowing them to pivot their strategies promptly, in a way that was unimaginable just a few years back. Additionally, by automating the sentiment analysis process, firms can save time and resources while minimizing the human error associated with manual analysis.
Automated News Sentiment Analysis
Automated news sentiment analysis is fundamental in grasping market sentiments. It revolves around extracting subjective information from various financial news sources to help forecast stock movements. Many organizations now rely on NLP frameworks to glean insights from textual data, which traditionally required significant human interpretative skills. Automation in this domain allows for:
- Speed: Information can be processed in real-time, which is crucial in day trading scenarios.
- Consistency: The analysis remains uniform despite organizational changes or external influences that could affect human interpretation.
- Volume Handling: The sheer volume of financial news generated each day means that manual analysis is not only inefficient but also impractical.
For instance, a sentiment analysis tool may scan hundreds of thousands of articles daily, flagging those with a predominantly positive or negative tone. This kind of automated insight is potentially a goldmine for traders who act on public perception before it reflects in stock prices.
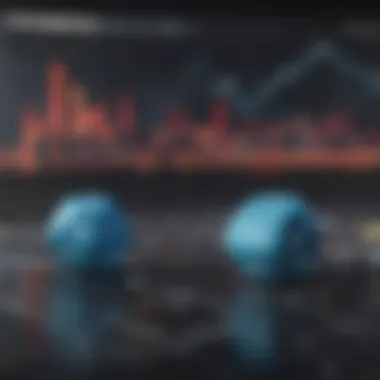
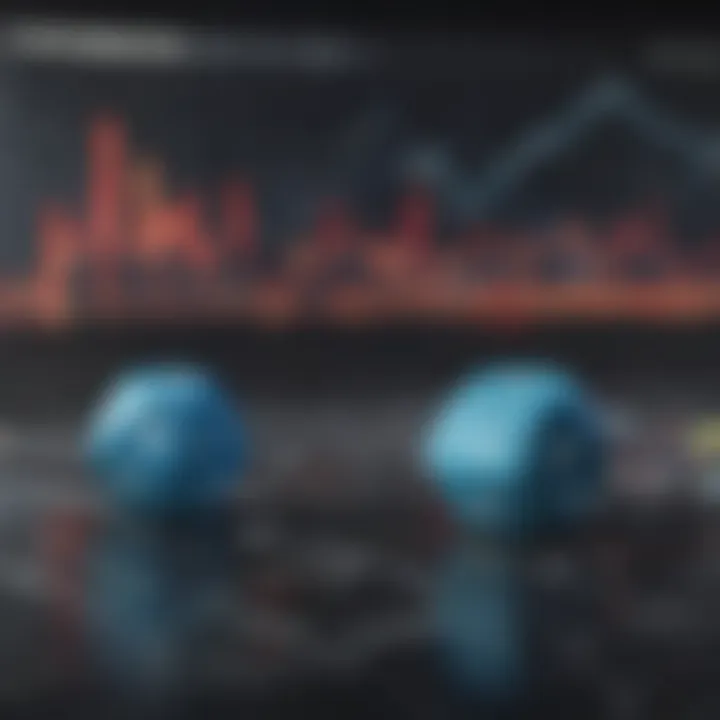
Impact on Stock Prices
The effects of natural language processing on stock pricing are pronounced. Investors have observed a correlation between news sentiment and stock movement. As NLP technology improves, this correlation may become even tighter. Positive sentiments, characterized by phrases like "record earnings" or "expanding market share," can trigger buying waves in stocks. Conversely, negative sentiments, marked by reports of layoffs or reduced forecasts, often lead to rapid sell-offs.
āUnderstanding the impact of language on market dynamics can revolutionize trading strategies.ā
The way financial news is communicated can sway public perception, and by extension, stock prices. Several key areas influence these impacts:
- Market Reactions: Investors often act on news before factual evidence is displayed in the market.
- Market Sentiment Trends: Sustained negative or positive sentiment in news can create momentum, affecting stock evaluations significantly.
- Herd Behavior: Understanding how sentiments shift among reported financial news can also give insight into potential herd behavior, leading to market bubbles or crashes.
In summary, NLP technologies aid in dissecting and interpreting financial news, providing critical insights that inform trading decisions and reflect on stock prices. As AI continues to evolve, the symbiosis between NLP and finance is bound to deepen, shedding more light on complex market dynamics.
Risk Management Through AI
Risk management is critical in the stock market, as it deals directly with protecting investments from potential losses. In todayās fast-paced trading world, investors face numerous uncertainties that can cause market fluctuations. Artificial Intelligence provides robust tools to aid in risk management, enabling both beginners and seasoned investors to navigate through market complexities more effectively.
One of the notable advantages of using AI for risk management is its ability to analyze vast data sets more efficiently than humans. It can process market data, economic indicators, trading volumes, and even social media sentiment at lightning speed. By leveraging machine learning algorithms, AI systems can identify patterns in historical data, which helps in predicting future market behavior. This predictive power is invaluable for anticipating potential downturns, allowing investors to act preemptively.
Furthermore, AI systems continuously learn and adapt. Unlike traditional methods that rely on fixed models, AI algorithms evolve with new data, ensuring that the risk assessment stays current. This adaptability is essential in a market that can change in the blink of an eye, keeping stakeholders one step ahead.
Identifying Risk Factors
Identifying risk factors is the bedrock of effective risk management through AI. Intelligent algorithms sift through mountains of data to highlight what poses the most significant threats to an investment. Key elements include market trends, volatility, and economic indicators.
The AI process typically involves:
- Data Collection: AI gathers data from various sources, such as financial reports, news articles, and social media.
- Risk Assessment Models: Machine learning models categorize and quantify risks based on data analysis. They might assess historical price movements, correlations, and external market conditions.
- Real-Time Monitoring: Investors receive timely updates on potential risks, allowing them to adjust their strategies as needed.
For example, if a specific stock starts showing erratic price movements correlated with negative news sentiment, the AI can flag this as a risk factor. Such proactive identification can make the difference between a preserved investment and substantial losses.
Portfolio Optimization
Portfolio optimization is another area where AI shines, enabling a balanced approach to diversifying an investment portfolio. With multiple assets, an investor must ensure they're not overexposed to any single risk factor. AI algorithms utilize complex computations to assess the combinations of assets and manage risk while aiming for maximum returns.
Hereās how AI aids in optimizing a portfolio:
- Diversification: AI can analyze how different asset classes move in relation to each other, guiding investors to create a diversified portfolio that minimizes risk without sacrificing potential returns.
- Continuous Rebalancing: Unlike a static portfolio that remains unchanged, AI allows for continuous monitoring and adjustment based on market conditions, ensuring the portfolio aligns with the investorās risk tolerance and goals.
- Simulation Models: AI runs multiple scenarios to simulate how a portfolio would perform under various market conditions. This feature aids in understanding potential outcomes and assists in making informed decisions.
Ethical Considerations of AI in Stock Markets
The advent of artificial intelligence in stock markets brought with it a slew of benefits that can not be ignored, such as enhanced trading strategies and superior market predictions. Yet, lurking beneath the surface are profound ethical considerations that must be addressed to ensure a fair and just financial ecosystem. The intersections of advanced technologies with investment decisions are not just technical matters; they encompass societal impacts that ripple through economies and communities. Thus, this section delves into the ethical considerations, particularly focusing on bias and transparency.
Bias and Discrimination in Algorithms
AI operates on algorithmsāmathematical equations designed to make decisions based on data inputs. However, it is critical to acknowledge that these algorithms are only as good as the data they are fed. If the datasets include historical biases, the algorithms can perpetuate or even exacerbate existing inequalities. This means a trader using an AI system that analyzes historical stock performance might unintentionally favor certain demographics over others, leading to discrimination in investment opportunities.
- Underlying Bias: Data can be skewed due to various factorsāhistorical unequal growth patterns among industries or the diversity of the data sources. This could cause algorithms to reflect bias prevalent in past trading behaviours.
- Performance Metrics: Some metrics commonly used in evaluating algorithms, such as Sharpe Ratios, could be more beneficial for certain groups over others due to inherent biases in the historical data.
The social ramifications of biased AI can be grave. Imagine an investor who relies on an AI-driven advisory service. If the service is biased, decisions made could disproportionately disadvantage certain groups, raising questions about fairness and equality in financial markets. Addressing bias isn't just about better performance but about providing a level playing field in investing.
Transparency and Accountability
A second ethical dimension revolves around transparency and accountability in AI systems used in stock markets. As algorithms dictate substantial financial decisions, it becomes crucial that these systems are not viewed as black boxes, where users have no clue how outcomes are generated. This lack of clarity can foster mistrust and confusion.
- Algorithmic Opacity: Investors need to understand how decisions are reached. Without transparency, itās too easy for algorithms to be manipulated or produce questionable results out of sight.
- Holding Entities Responsible: If an AI system malfunctions or produces faulty advice leading to significant financial loss, it raises fundamental questions about who is accountable. Is it the developer of the software, the financial institution using it, or the investor who made the decision based on it? Clear guidelines must be established to ascertain responsibility.
To enhance trust among users and stakeholders, the industry may consider adopting standardized practices that promote transparency. The incorporation of explanations along with algorithmic decisions not only builds confidence but also facilitates a dialogue on the ethical use of AI in finance.
"Transparency and accountability are not mere add-ons, but foundational elements that can shape the future of AI in the stock market."
These ethical considerations underscore the urgent need for regulators, developers, and practitioners to collaborate to ensure AI delivers benefits equitably while minimizing negative impacts. As we continue to integrate AI into stock trading, it is vital to reflect on its broader implications for society.
Challenges and Limitations of AI Technologies
The integration of artificial intelligence in the stock market brings impressive potential, yet it is not without its share of hurdles. Understanding the challenges and limitations of AI technologies is crucial for grasping their true effectiveness in financial markets. The interplay of these challenges impacts not only algorithm performance but also broader investment strategies and risk management. As investors or financial enthusiasts, recognizing these limitations can refine decision-making processes and improve strategies.
Data Quality and Integrity
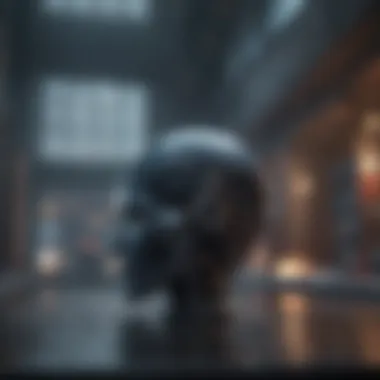
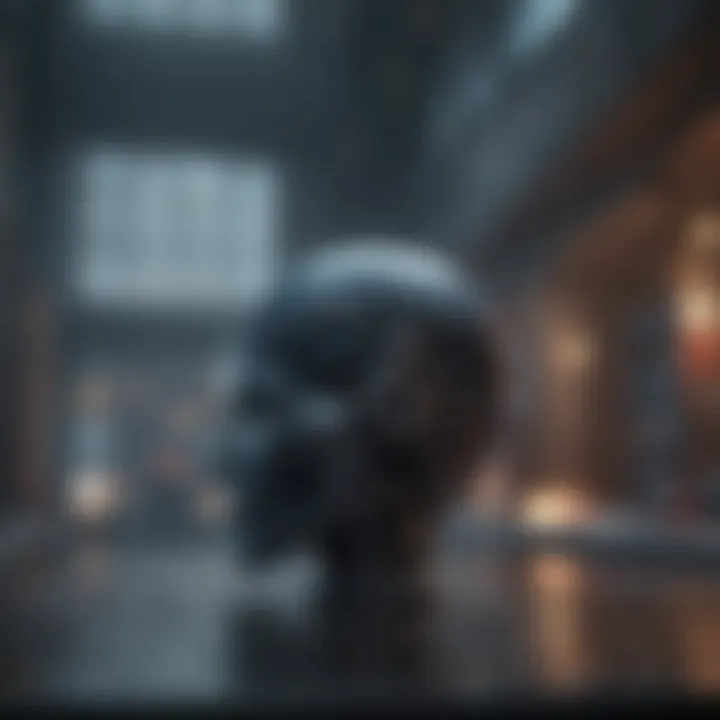
At the heart of any AI system lies data. Specifically, the quality and integrity of that data can make or break an AI initiative. Faulty data may lead to erratic predictions and misguided trading strategies. For instance, if an algorithm relies on historical price data that is corrupted or incomplete, the resulting models can amplify errors. This could, in the worst case, translate into significant monetary losses.
Moreover, fluctuations in data sources can muddy the waters. Relying heavily on data from one source can create biases. In general, diversity in data sources is often seen as a safeguard. A well-rounded datasetāincorporating various industries and market conditionsācan provide a fuller picture, allowing for more robust analysis and better insights.
- Ensure reliable sourcing: Look towards reputable financial institutions for data.
- Implement thorough cleansing processes: Regularly audit data streams for inaccuracies.
- Diversify datasets: Use multiple data sources to minimize bias and inconsistency.
"Good data is like good oil; it powers your machine and keeps it running smoothly."
AI systems also require ongoing maintenance to adapt to market shifts. Market crashes, sudden economic changes, or unprecedented global events can render older models ineffective. Essentially, the data must be not only high-quality but also relevant and up-to-date. If the training data does not reflect current market dynamics, an AI system can quickly become obsolete.
Regulatory Barriers
Navigating the regulatory landscape presents another top-tier challenge for AI applications in the stock market. Regulatory bodies constantly evolve their frameworks to keep pace with rapid technological advancements. This constant shifting creates uncertainty for investors aiming to harness AI.
The nuances of compliance can be daunting. For example, algorithms that operate under a premise of high-frequency trading might raise red flags regarding market manipulation or insider trading. Hence, traders must ensure that their strategies conform not only to local regulations but also to those of markets they engage in globally.
Considerations include:
- Licensing Requirements: Some jurisdictions may require special licenses for algorithmic trading, adding bureaucratic hurdles.
- Data Privacy Laws: Regulations like GDPR in Europe mandate a strict approach to personal data, which can affect how financial data is collected and utilized.
- Transparency vs. Competitive Edge: Striking the right balance between maintaining proprietary algorithms and being transparent enough to satisfy regulatory scrutiny is a tightrope that traders must walk carefully.
In summary, while AI has the potential to fundamentally alter the stock market landscape, it must navigate significant hurdles related to data integrity and regulatory compliance. Addressing these challenges can lead to more effective strategies and contribute to a more stable market environment.
Future Trends in AI and Stock Trading
The pace of change in the financial markets is accelerating, and artificial intelligence is at the forefront of this transformation. Understanding future trends in AI and stock trading is crucial. Itās not just about predicting outcomes but reshaping how investments are made altogether. As algorithms become more sophisticated, and data continues to grow, the integration of AI into trading strategies will only deepen.
Advancements in AI Algorithms
AI algorithms are evolving, making them more effective in processing vast amounts of data. The essence of these advancements lies in their ability to analyze patterns that humans cannot easily discern.
- Continual Learning: These algorithms can adapt based on feedback and new data. They don't just operate on static data sets; they learn continuously. This means that forecasts about market trends can improve over time, giving investors a sharper tool to leverage.
- Deep Learning: Techniques in deep learning have taken center stage. Neural networks now mimic the human brain's interconnectedness, allowing for more nuanced analyses. For investors, this translates to better predictions based on intricate datasets, such as historical stock prices combined with economic indicators.
- Predictive Analytics: Algorithms are now designed to not only react to market changes but to anticipate them. Predictive models utilize historical data to create simulations that help in forecasting future stock movements.
By employing these advancements, investors can harness data like never before, turning the complex into actionable insights.
Integration with Blockchain Technology
The intersection of AI and blockchain technology is a promising frontier for stock trading. For many, blockchain is synonymous with cryptocurrencies; however, its application extends far beyond just digital currencies. Hereās how these two technologies complement each other:
- Enhanced Security: Blockchain offers a decentralized ledger that is tamper-proof, making transaction records secure and transparent. When combined with AI, firms can ensure that their trading strategies and transactions are robust against fraud.
- Smart Contracts: These self-executing contracts with the terms directly written into code can streamline trading processes. An AI system can analyze its environment, and when certain conditions are met, trigger a trade automatically. This reduces the time frame from decision to execution, which is critical in high-frequency trading scenarios.
- Real-Time Data Sharing: Blockchain can enhance data sharing capabilities between traders and financial institutions. AI can employ this stream of real-time data for immediate analysis, thus improving decision-making processes.
The fusion of AI and blockchain not only stands to optimize stock trading but also to innovate how transactions are facilitated in essence.
"In a world driven by data, the union of AI with blockchain represents a significant leap towards transparency and efficiency in financial markets."
By examining these emerging trends, both novice and seasoned investors can better position themselves in the fast-evolving landscape of finance.
Concluding Remarks
In the rapidly evolving landscape of financial markets, artificial intelligence stands as a pivotal force shaping the ways investors analyze and respond to market dynamics. The discussions encapsulated in this article provide a thorough examination of the ways AI is interwoven into stock trading, risk management, and the broader financial ecosystem.
The importance of summarizing the key points rests not only in reinforcing the knowledge conveyed but also in drawing connections that aid in comprehension. By revisiting the various applications of AIāsuch as algorithmic trading, market analysis, and risk managementāinvestors can better appreciate how these technologies facilitate informed decision-making.
Key benefits include:
- Enhanced predictive capabilities, allowing traders to anticipate market movements more accurately.
- Reduction of human error, as AI algorithms can process vast amounts of data swiftly and systematically.
- Increased efficiency in trading strategies, enabling quicker responses to market shifts.
Yet, as AI continues to innovate within the stock market, one must also consider its limitations. Various challenges, such as data quality, transparency, and ethical concerns, loom large. Without addressing these issues, the potential for unintended consequences increases, underscoring the necessity for a balanced approach in embracing AI technologies in finance.
"AI is a tool. Itās not the panacea; rather, itās one of the many tools investors can utilize in their quest for insightful analysis and effective trading."
Summarizing Key Points
Throughout this article, we've unpacked multiple dimensions of AIās role in stock trading, emphasizing the significance of:
- Applications of AI in algorithmic trading and market analysis.
- The advancements in machine learning that refine pricing models.
- The critical ethical considerations regarding bias and transparency that every stakeholder should be mindful of.
- The challenges still facing the integration of AI technologies, such as regulatory hurdles and ensuring data integrity.
These discussions are essential not only to grasp the current capabilities of AI in finance but also to prepare for its ongoing evolution. Investors who stay informed about these developments are likely to navigate the stock market with greater success and foresight.
Outlook on the Role of AI
Looking ahead, the future of AI in stock trading promises to be both exciting and complex. Emerging trends, such as the sophisticated refinement of AI algorithms and their anticipated integration with technologies like blockchain, set the stage for what can be achieved in finance.
Some potential trends to observe include:
- AI Advancements: Expect algorithms to become more sophisticated, offering insights beyond basic market patterns, enabling proactive risk mitigation.
- Blockchain Integration: As both AI and blockchain mature, their combined use could lead to unprecedented levels of trust and transparency in financial transactions, revolutionizing how trades are executed.
- Increased Personalization: AI can potentially transform customer experiences by providing tailor-made investment strategies, based on individual risk profiles and market conditions.